Prototype Your GenAI Idea in Less Than 20 Days
Test your GenAI concepts quickly and affordably with our POC package—working prototypes in less than 20 days for under $20,000.
Get started todayWhy Choose First Line?
We are experienced and ready to answer your questions. Here are the most common things leaders ask us:
What if my team doesn’t fully understand RAG architecture?
We understand your concern. RAG is a complex field, but our team can provide hands-on guidance and support throughout the process. We’ll break down the complexities, guide your team through each step, and start with small, low-risk projects. Nobody needs to be an expert from the beginning. We’ll also conduct a thorough assessment of your existing systems to develop a phased integration plan that minimizes disruption.
What if our data isn’t in great shape? Will it hinder the performance of the RAG model?
Data quality is crucial, but we specialize in improving it. We’ll prioritize data sources based on impact and implement automated data-cleaning processes. We’ll also fine-tune the model to adapt to improving data quality, ensuring incremental improvements.
How can we manage the costs of the infrastructure needed for RAG?
We can start with a minimal, proof-of-concept model to minimize upfront expenses. As you see results and feel more comfortable, we can scale the infrastructure incrementally. We’ve helped companies implement RAG within various budget ranges and will ensure a manageable and scalable cost structure.
Now that we have implemented RAG, how do we know it is performing correctly?
As GenAI continues to evolve, rigorous evaluation is crucial to mitigate potential risks and ensure continued client satisfaction. Unlike traditional software, GenAI models can produce unpredictable, harmful, or biased outputs if not carefully designed and tested. We believe a that multi-faceted approach to evaluation is essential to identify, measure, and mitigate these risks, ensuring continued client satisfaction and reliable support.
RAG Implementation Explained
From a client perspective, RAG works by allowing employees and clients to search for information within the enterprise’s vast dataset and generate relevant content based on their queries. This streamlined process ensures quick access to accurate and up-to-date information.
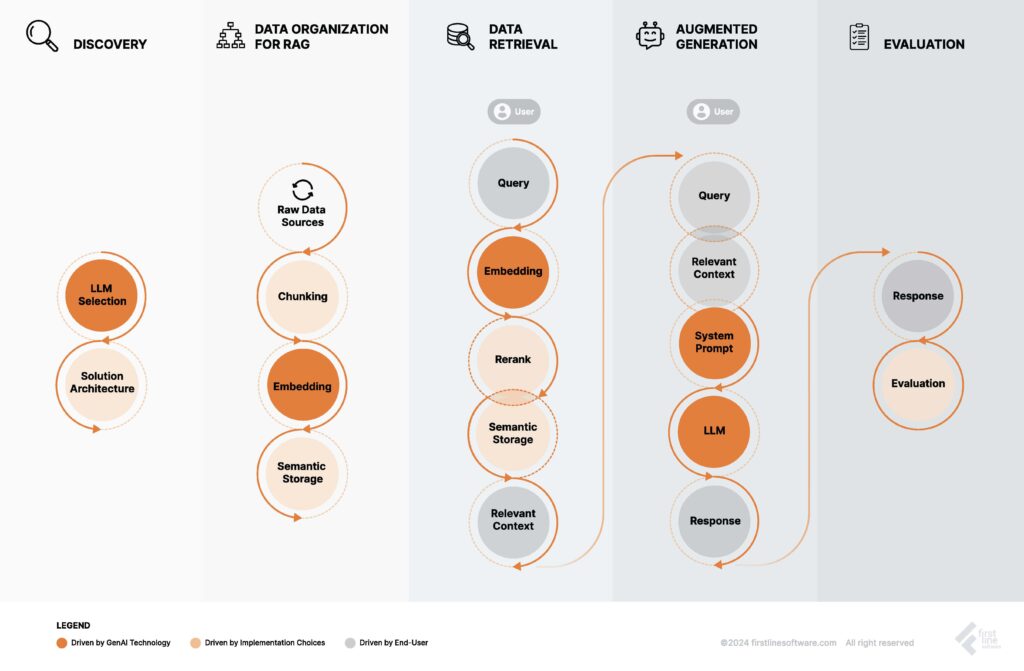
RAG Implementation Services
Retrieval-Augmented Generation (RAG) is a groundbreaking AI technique that combines the power of retrieval-based systems with generative models. This hybrid approach enables the generation of highly accurate and contextually relevant content, making it a valuable tool for various applications.
Discovery:
During the discovery period, we provide a comprehensive analysis of evaluated LLMs, including recommendations and justifications, as well as a detailed overview of the proposed system architecture, outlining components, data flow, and interactions.
Data Organization:
Data from various sources, including text, documents, and databases, is collected, extracted, cleaned, preprocessed, indexed efficiently for retrieval, and stored in a suitable format.
Data Retrieval & Augmented Generation:
The system processes user queries by converting them into numerical representations, searching through indexed data using semantic search, and combining retrieved information with the query to form a relevant context. We meticulously integrate carefully selected components into a cohesive system, ensuring its optimal performance, accuracy, and user-friendliness.
RAG Evaluation:
We employ a comprehensive approach to assess RAG models, identifying and mitigating biases, hallucinations, and other risks. By continuously evaluating, optimizing, and managing datasets, we ensure the reliability, accuracy, and safety of our clients’ RAG systems.
Breaking It Down In More Detail
Discovery
LLM Selection: A suitable language model is selected based on the specific requirements of the RAG system.
Solution Architecture: Appropriate components, such as a language model, retrieval system, and data storage, are selected for the RAG system.
Data Organization for RAG
Raw Data Sources: The process begins with identifying and gathering relevant data sources that will form the knowledge base for the RAG system.
Chunking: Breaking down large amounts of data into smaller chunks for efficient processing.
Embedding: The query is converted into a numerical representation (embedding) that can be compared to the indexed data.
Semantic Storage: The system stores and retrieves information based on its semantic meaning rather than its exact keywords or phrases
Data Retrival
Query: A user submits a query or question to the RAG system.
Embedding: The query is converted into a numerical representation (embedding) that can be compared to the indexed data.
Reranking: The results are ranked based on relevance.
Semantic Storage: The system searches through the indexed data using semantic search techniques to find the most relevant information based on the query’s meaning.
Relevant Context: The retrieved information is combined with the original query to form a relevant context.
Augmented Generation
Query: A user submits a query or question to the RAG system.
Relevant Context: The retrieved information is combined with the original query to form a relevant context.
System Prompt: A prompt is generated based on the query and context, providing the LLM with the necessary information to generate a response.
LLM: A large language model (LLM) is used to generate a response based on the prompt and the retrieved context.
Response: The system provides the user with a response.
Evaluation
Response: The system provides the user with a response.
Evaluation: The system reviews the system’s performance for future queries.
How We Drive Success
RAG for Enterprises:
For RAG implementations, we utilize the latest open-source frameworks and leverage Azure’s infrastructure to access cutting-edge language models.
Accelerated GenAI Excellence
We embrace a GenAI-first engineering culture. We leverage GenAI to streamline our software development lifecycle, delivering exceptional solutions faster and more efficiently.
Engagement Models
Flexible Delivery Centers
Expand your team while benefiting from shared business knowledge, aligned goals, and expectations.
Dedicated Delivery Centers
Gain a fully scaled team tailored to meet your specific solution requirements.
Turnkey Projects
Leverage our expertise across the entire development cycle to successfully deliver your projects.
Technical Expertise Engagements
Boost your team’s capacity, skills, and experience with our specialized expertise.
First Line Software at a Glance
0+
Years of Technology Experience
0s
Projects Delivered
0s
Satisfied Clients
0%
Client Retention Rate
Clients Love Us
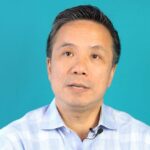
Qi Li
Physician Executive, Product Development At Intersystems
“They are very good at understanding the requirements but more importantly they can think about the future requirements and future proof your project.”
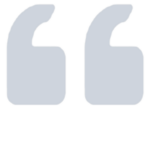
Executive
Real Estate Appraisal Company
“First Line Software is paying attention and proactively managing the project with us while doing extra things that go above and beyond what we expect.”
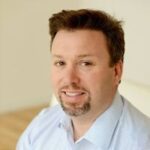
Jay Thomas
Chief Strategy Officer, Triptych, USA
“First Line Software has a large team, and because of the breadth of services, they can give you that flexibility to work on other projects. Adding another technology to your stack, for example, is one way they could support you.”
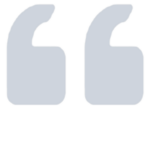
Technology Project Manager,
Sandy Alexander, New York
“I’ve worked with other developers, but I found that the First Line Team is very knowledgeable and helpful. I would totally recommend them to anyone who’s looking into their services.”