4 Ways You Can Use Machine Learning in Manufacturing
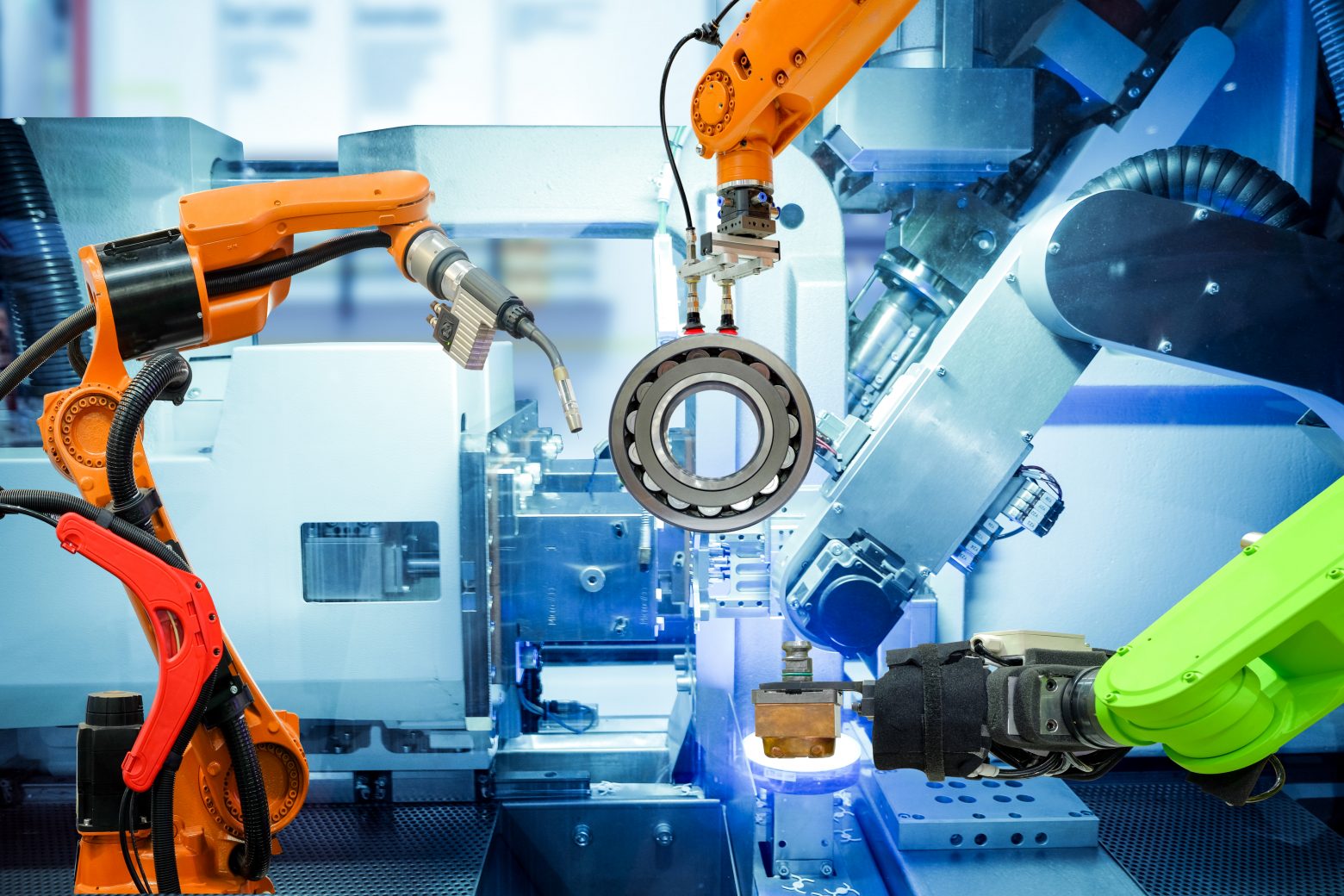
Machine learning is at the heart of many innovative artificial intelligence technologies. Programs developed with ML can predict equipment failures, customer behavior, and make logical and analytical decisions in some circumstances almost like humans. Let’s take a look at how companies use machine learning in manufacturing with a few real-word examples, and explore 4 ways you can apply this technology to your manufacturing process.
The ability of companies and industries to stay ahead of their competition in the near future will undoubtedly depend on whether they can successfully carry out some form of digital transformation. Internet-of-Things (IoT) technologies and Machine Learning will play a key role in these changes, especially in the manufacturing sector. But not all companies have the experience and resources to successfully implement complex IoT and ML projects.
The full-scale implementation of Machine Learning technologies is a complex integration project that involves merging large amounts of data. This data must be continuously obtained from a geographically distributed fleet of sensors, controllers, metering devices, smart devices, and other equipment; all efficiently stored and processed to extract the maximum technological and economic benefits from the data. The greatest challenge here is to ensure the integration and linking into one system of cloud technologies, databases, analytical algorithms, various types of sensors, interfaces, data formats, mobile devices, and communication networks. However, few enterprises have the experience, knowledge, and specialists to implement such projects from scratch and within a reasonable amount of time.
Since time is always of the essence, given the speed with which digital transformation is currently unfolding around the world, any delay in digitalization can quickly lead to a deterioration in a company’s competitive position. Most of these companies will have to seek support from hardware and software vendors, consulting firms, and platform and IT service providers to implement IoT and Machine Learning solutions.
Machine Learning in Manufacturing: Production, Downtime Minimizing, and Accidents
In the manufacturing sector, ML can be applied to deliver real business benefits such as:
1. Predictive diagnostics
One of the practical directions for using machine learning platforms has become predictive diagnostics of equipment and products, which makes it possible to predict the onset of an emergency situation in advance based on analysis and monitoring of its current state and prediction of failures. As a result, the company can prevent the problem or mitigate the adverse effect.
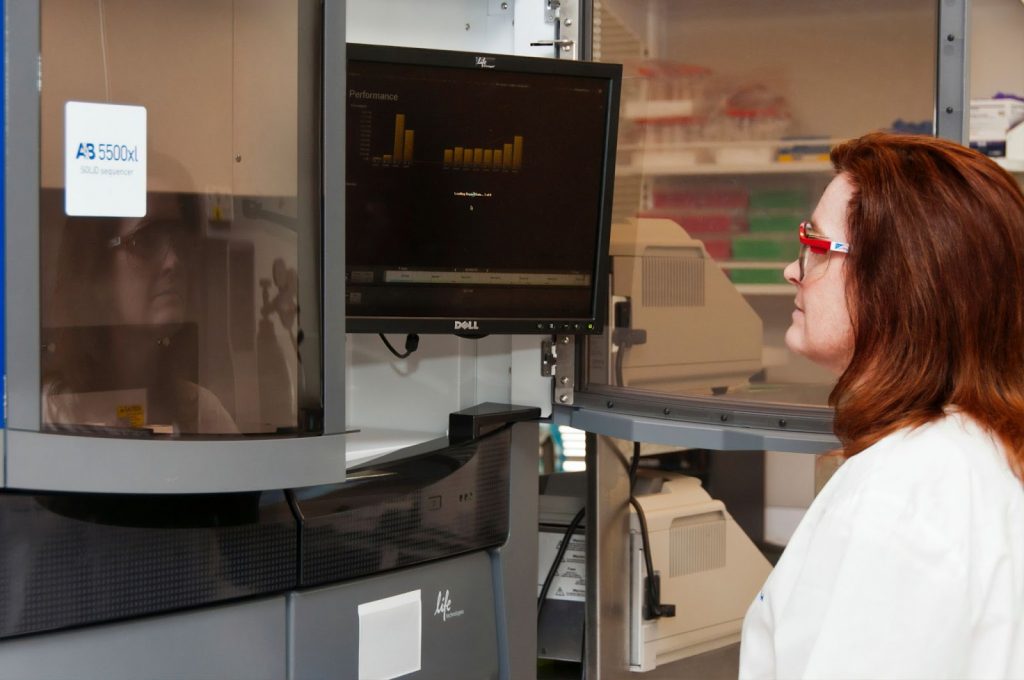
Machine learning platforms have all the necessary tools for building predictive diagnostic systems. This includes a set of algorithms and libraries for building these models using deep machine learning, neural networks, and mathematical models. Ready-made analytical modules can be quickly configured and connected to industrial data sources that display the operation of industrial systems.
Data is collected from equipment sensors that indicate where failures are likely to occur. Using this information, technicians can predict when and why downtime will happen, and how to avoid it.
For example, if the temperatures in a foundry rise too quickly, it may lead to other equipment breaking down. Algorithms of ML that have been implemented in the system will notify the engineers of the increase in temperature, and they can prevent the problem before it becomes one.
In another industry, – Oil and gas manufacturers use the Industrial Internet of Things and Machine Learning to avoid downtime in mining operations. General Electric (GE), for example, has implemented various digital industrial solutions. The company’s platforms collect data on the state of oil production and then schedule diagnostic checks to help identify problems before they happen. In this approach, gas production is increased by 2-5%, and maintenance costs are reduced by 10%.
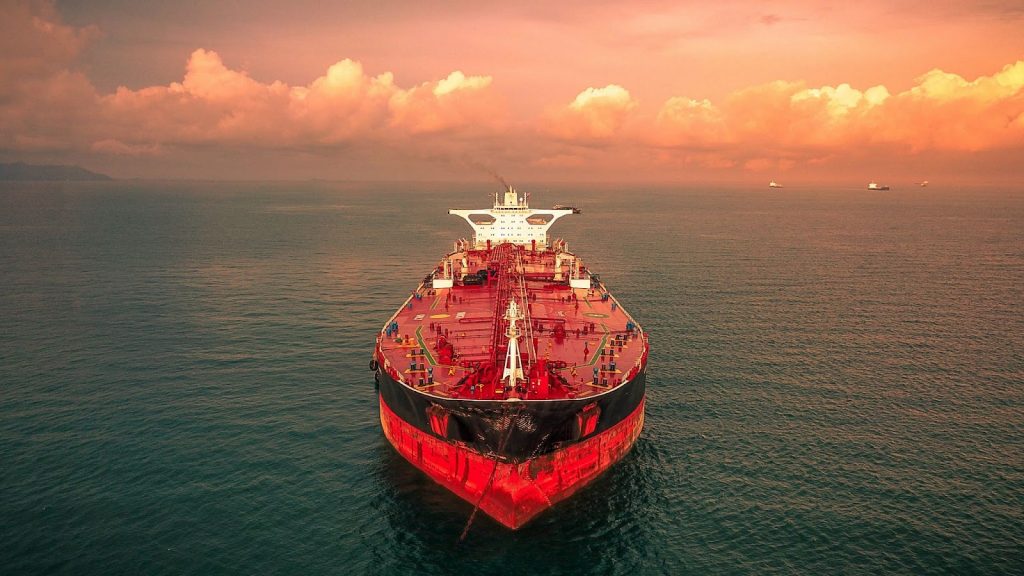
2. Production management systems
Sensors and machine learning solve not only narrow tasks like preventing breakdowns, but also manage the entire production:
- It can reduce the percentage of defective parts by analyzing why the defect occurs and how to avoid it
- Use fewer materials for production, which means lower costs
- Monitor the equipments condition, optimize its efficiency and workload
- Automate individual stages of production
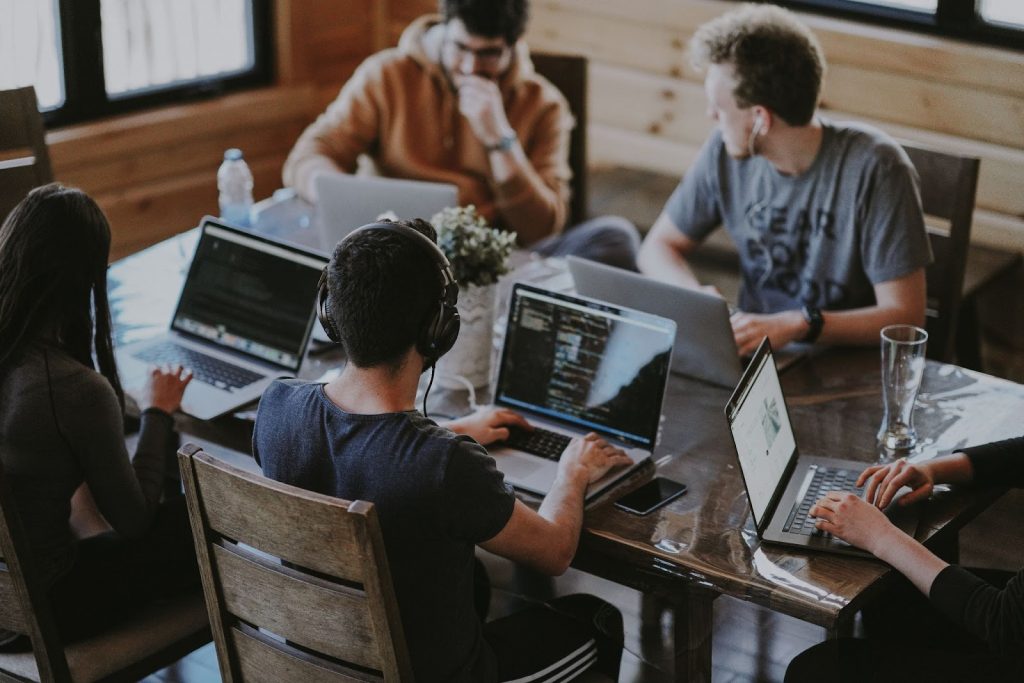
For example, the First Line Software team developed an integrated IoT platform that collects, stores, and processes data from equipment for one of our clients. We modernized the IT infrastructure for a company that provides services for managing production assets by creating a customizable, scalable application that reduces the cost and time of aircraft equipment maintenance. Chips are installed in equipment that requires regular maintenance and monitoring of service life. The chip’s memory contains general equipment characteristics, movement data, repairs, etc. The data is read by a scanner connected to a smartphone. To work with these devices, a special mobile app was developed that allows entering information into the system directly at the site. Through the app, an employee creates requests for repair or replacement. The repair service sees the request in the system and processes it. The platform significantly reduces the time to check an object from several hours to half an hour, minimizes the number of errors in reports, and allows for quickly replacing and repairing equipment.
3. Identification of security threats
Machine learning helps to make production safer: to detect minor changes in the operational process and rapidly notifying employees of a potential accident.
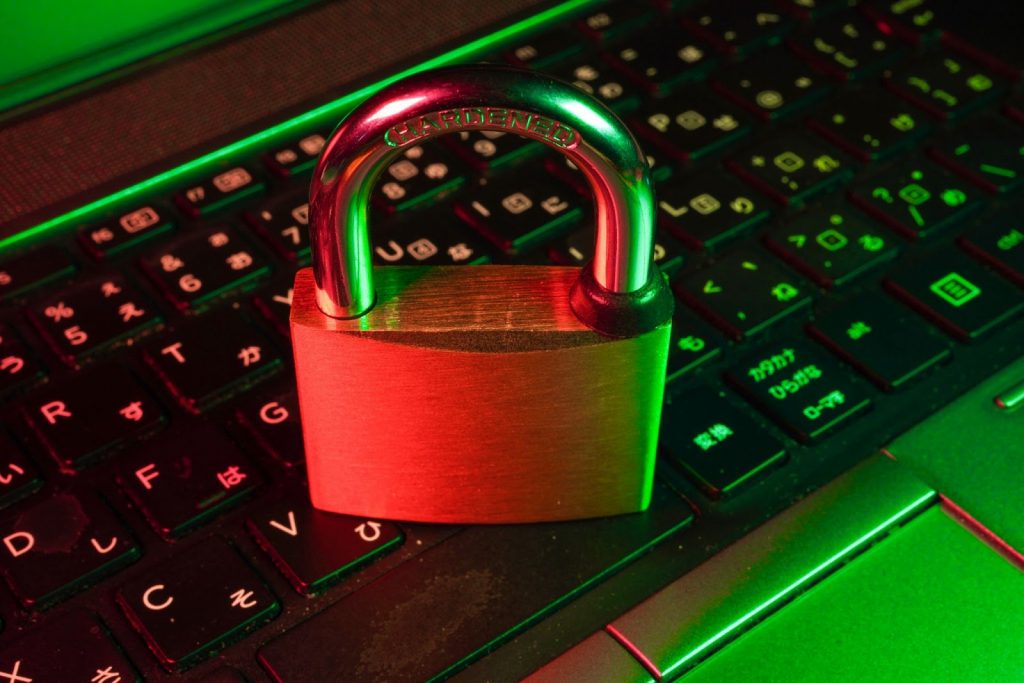
The global company, Shell, uses machine learning, neural networks, and IoT to automatically detect security threats and alert employees about them. In addition, Shell also uses machine learning to optimize their production and mining operations.
4. Exploration of new fields
One of the main problems of the oil, gas, and mining industry is the difficulty in discovering new fields. Machine learning helps speed up this process. Based on data from past fields, artificial intelligence builds models that predict with high accuracy where to search for new fields of gas or ore.
Artificial intelligence and machine learning provide the company with information about:
- 3D rock and void structure;
- Elemental, chemical, and mineral composition of the reservoir components;
- Reservoir fluid properties;
- The results of computer modeling of reservoir properties and other physical properties of rock and reservoir fluids;
- Data of the results of laboratory experiments.
Oil companies operate digital labs where reservoir samples are analyzed using machine learning technologies. Algorithms simulate conditions where the sample is taken from and help create a digital twin of the oil deposit. With its help, mineral reserves are assessed and involve an individual approach to development. This allows companies to increase the extraction of minerals from a particular deposit by 1.5-2 times, as well as look for new ones.
For example, these methods increase General Electric (GE) production from a well, with the succeeding in increasing production by 2-5% from the optimization exercise.
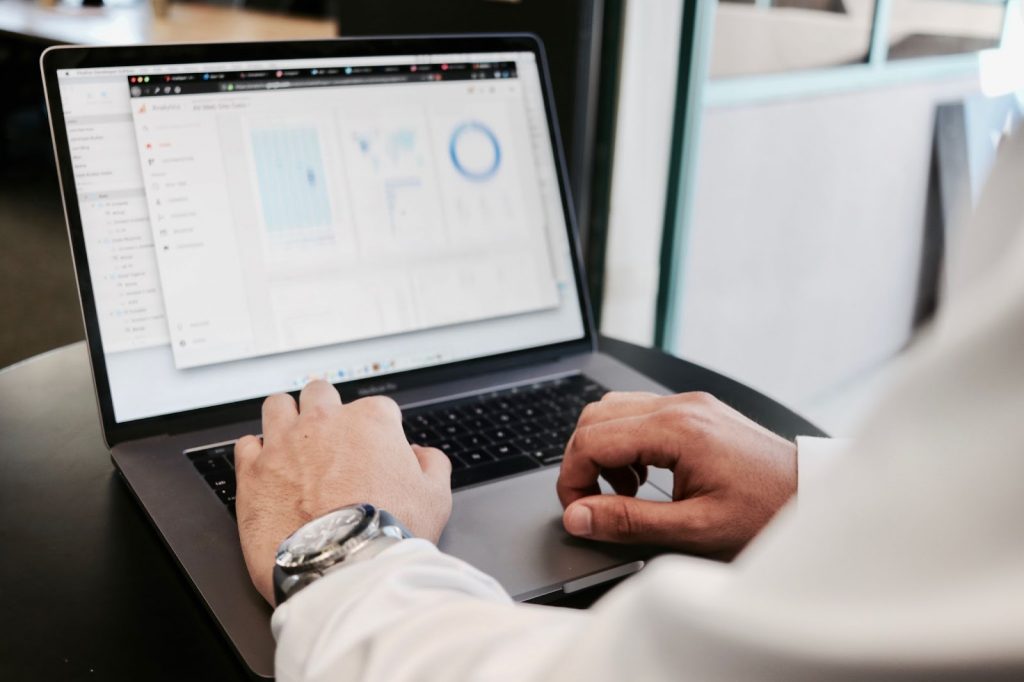
The Digital Age Needs Digital Manufacturing. First Line Software is Already with You!
Due to the rapid development of information and communication technologies, as well as the steadily emerging demand for ML solutions, dozens of IoT platforms enter the market every year. First Line Software is well-positioned to be at the forefront of Machine Learning, enabling customers to transform ML data into useful business results. We are opening up new opportunities in the digital age for global customers and partners. Contact our team to discuss the opportunities!