Clinical Decision Support Systems 101: All You Need To Know
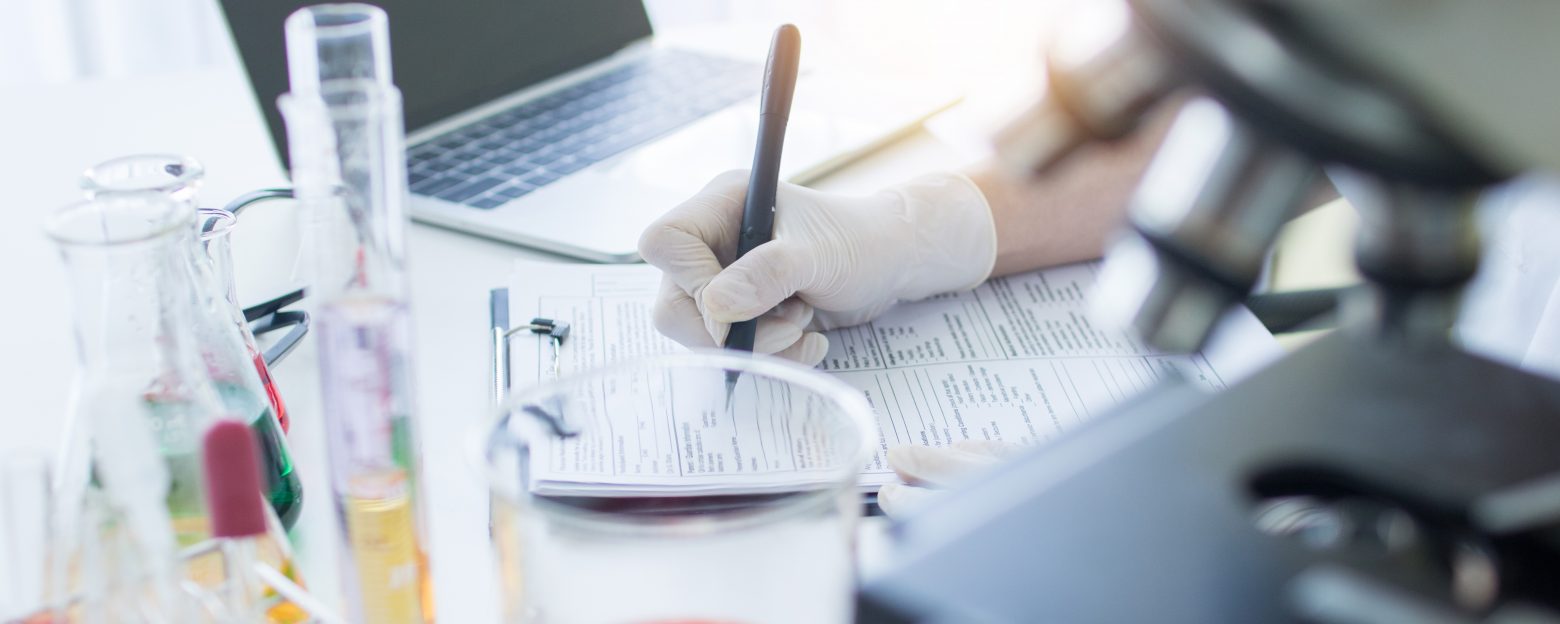
How can clinical decision support systems deliver patient safety and care quality improvements while ensuring health IT usability?
What is a clinical decision support system (CDSS)?
A clinical decision support system (CDSS) is a computer-based program that analyzes EHR data to provide prompts and reminders to help providers implement evidence-based clinical guidelines at the point of care.
Clinical decision support systems link health observations and health knowledge to inform health decisions for improved health management by clinicians. CDSS is a great example of how artificial intelligence can be used in medicine.
The most recent methodology for using her CDSS in support is that the clinician interacts with her CDSS and uses her own knowledge and her CDSS’s knowledge to analyze patient data better than either a human or her CDSS could do alone. means to use both. CDSS is usually suggested for the clinician to consider, and the clinician is expected to extract useful information from the results presented and reject her CDSS proposal, which is erroneous.
How to design a clinical decision support system depends on the goals of CDSS. Let us dive in.
How does a clinical decision support system work?
- Diagnosis decision support system (DDSS).
Unfortunately, DDSS has not (yet) made as much of an impact as other types of her CDSS. This is particularly true of physicians’ negative perceptions and biases, poor accuracy (often due to data availability gaps), poor system integration (often due to data availability gaps), and manual Poor system integration that requires data entry. The latter is improved by her better EHR integration and standardized vocabulary.
- Case-based reasoning system (CBR).
CBR systems use previous case data to help determine the appropriate dose of radiation and optimal beam angle for radiotherapy treatment of brain cancer patients. A medical physicist and an oncologist then review the recommended treatment regimen to determine its feasibility.
- Triage out-of-hours management.
DDSS can help triage out-of-hours conditions by suggesting appropriate next steps for the patient (for example, call an ambulance or see a GP the next business day).
Knowledge or non-knowledge based?
- Knowledge-based CDSS
Most CDSSs consists of three parts: a knowledge base, an inference engine, and a communication mechanism.
A rule (IF-THEN statement) is created, and the system takes the data and evaluates the rule to generate an action or output7. Rules can be established using literature-based, practice-based, or patient-based evidence.
A knowledge base contains associations between rules and compiled data, most commonly in the form of IF-THEN rules. If this is a drug interaction determination system, the rule is that if drug X is taken and drug Y is taken, warn the user.
A separate interface allows advanced users to edit the knowledge base to keep it up to date with new solutions. The inference engine combines knowledge base rules with patient data. Communication mechanisms allow the system to display results to the user and provide input to the system.
- Non-knowledge-based CDSS
CDSSs that are not knowledge-based still require a data source but are not programmed to follow the knowledge of medical professionals but rather use artificial intelligence (AI), machine learning (ML), or statistical pattern recognition. Decide.
A CDSS without a knowledge base uses a form of artificial intelligence called machine learning. This allows computers to learn from past experiences and find patterns in clinical data.
However, most clinicians do not directly use machine learning for diagnosis, reliability, and accountability because machine learning-based systems cannot explain the reasons for their conclusions. Nonetheless, they serve as a post-diagnostic system for suggesting patterns that can be further investigated by clinicians.
Evidence of effectiveness
As mentioned by the CDC, the evidence for the effectiveness of CDSS is very strong. Research studies investigating CDSS have high internal and external validity, the Community Prevention Services Task Force has concluded that CDSS is effective, and CDSS studies have been replicated with positive results. I’m here. Guidance on how to implement CDSS is available from several sources.
Systematic Reviews of CDSS
A 2005 systematic review concluded that CDSS improved physician performance in 64% of studies and improved patient outcomes in 13% of studies. CDSS features related to improved physician performance included automatic electronic prompts rather than requiring user activation of the system.
A 2005 systematic review found that “decision support systems significantly improved clinical practice in 68% of studies.” CDSS features associated with success include integration into clinical workflows rather than separate logins or screens, electronic templates rather than paper-based, providing decision support at the time and place of treatment rather than previously, and treatment recommendations.
Functions and advantages of CDSS
Patient safety:
- Preventing drug safety & prevention of medication errors.
- Prescribing, transcribing, dispensing, administering – everything is computerized and occurs within a connected system. In this case, you can use electronic drug dispensing systems (EDDS).
- Reminder systems for medical events, and not just those that are medication related.
Clinical management:
Need help following clinical guidelines? You can choose rules to encode into CDSS: reminders for testing alerts to a specific protocol for the patients, managing patients in research or treatment, tracking orders, preventive care, follow-ups, and so on. This way, clinicians can also reach out to patients not following their treatment, needing follow-up appointments, or finding candidates for research.
Cost Regulation:
CDSS can be cost-effective for health systems, through decreasing inpatient length-of-stay; CPOE-integrated systems suggesting cheaper medication alternatives; clinical intervention; reducing test duplication; suggesting cheaper alternatives to drugs, or conditions that insurance companies will cover.
Administrative functions:
CDSS can improve the quality of clinical documentation. Documentation accuracy is important because it can directly aid clinical protocols.
Moreover, you get extra support for clinical and diagnostic coding, ordering of procedures and tests, and patient triage, and you can get suggestions for a refined list of diagnostics codes to aid physicians in selecting the most suitable one.
Also, we are now seeing diagnostic systems being developed with non-knowledge-based techniques like machine learning, which may pave the way for more accurate diagnosis.
Diagnostics support
Laboratory and pathology:
- Alerts and reminders for abnormal lab results.
- Extend the utility of lab-based tests for the purpose of avoiding riskier or more invasive diagnostics.
- Interpretation tool where a test’s reference ranges are highly personalized, for example, age, sex, or disease subtypes.
Patient-facing decision support:
- The patient is the data’s end user or ‘manager’. This is an excellent step toward patient-focused care, and CDS-supported PHRs are the ideal tool to implement shared decision-making between patient and provider. When connected to EHRs, PHRs can have a two-way relationship, whereby information entered directly by the patient can be available to their providers. Also, information in the EHR can be transmitted to the PHR for patients to view. (read more on patient empowerment in our article on Healthcare as a Service (HaaS)).
- Collect information from health devices and other wearables to create actionable insights for providers.
- Serve shared decision-making between patient and provider and interactive tools to make patients more knowledgeable/involved in their care.
Conclusion
CDSS have been shown to augment healthcare providers in various decisions and patient care tasks, and today they actively and ubiquitously support the delivery of quality care. CDSS continues to evolve in complexity through advances in AI, interoperability, and new sources of data.
Some applications of CDSS have more evidence behind them, especially those based on CPOE. Support for CDSS continues to mount in the age of the electronic medical record, and there are still more advances to be made, including interoperability, speed and ease of deployment, and affordability. At the same time, we must stay vigilant for potential downfalls of CDSS, which range from simply not working and wasting resources to fatiguing providers and compromising the quality of patient care. Extra precautions and conscientious design must be taken when building, implementing, and maintaining CDSS.