Five Reasons Why Your Clinical Analytics May Be Inaccurate
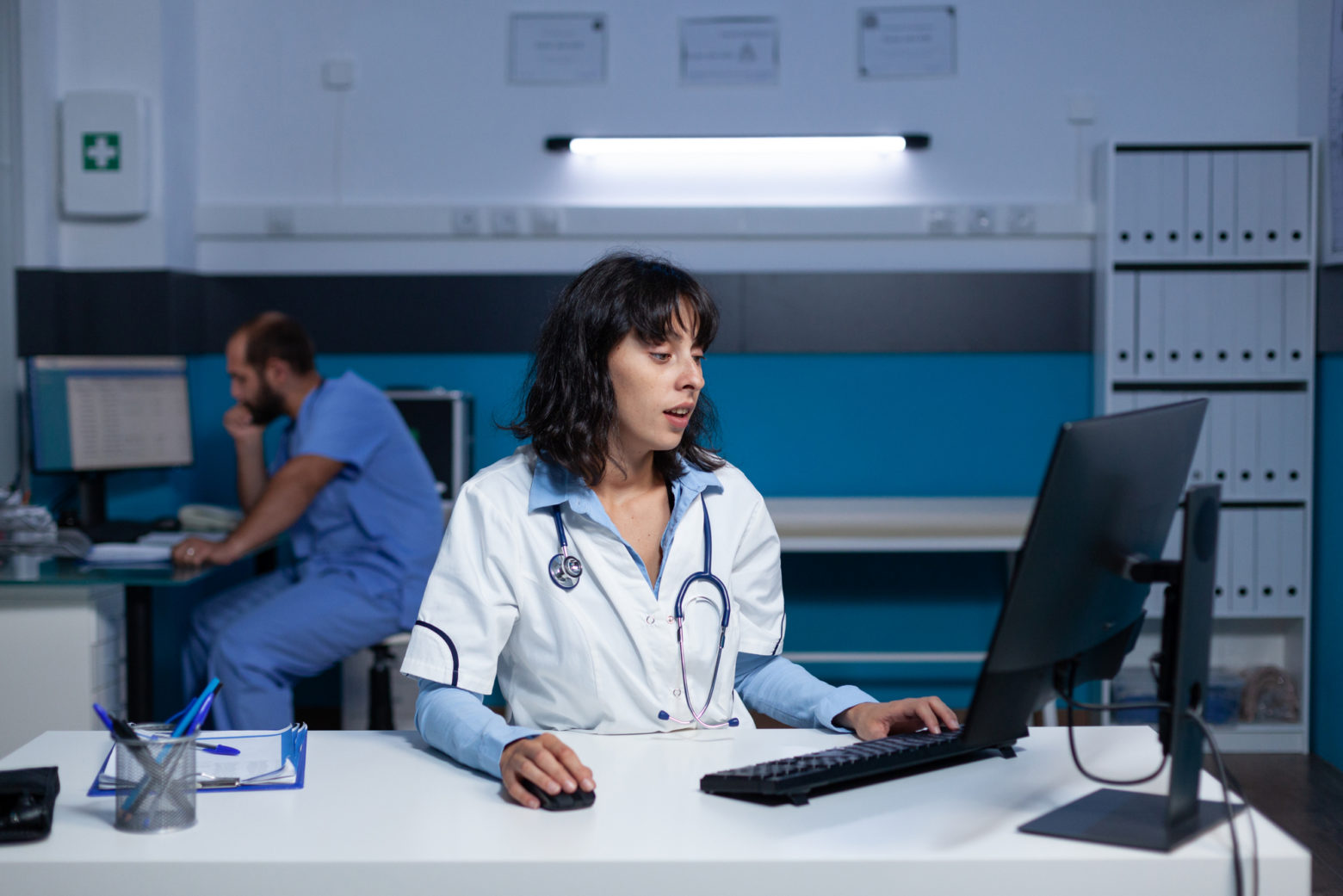
Or how to make your clinical data meaningful and usable. The adverse effects of clinical data quality on organizational performance and patient care are well documented and consolidated efforts for remediation are in progress.
Here at First Line Software we work and analyze the wealth of clinical data extracted and aggregated from EHRs and other systems. As part of these efforts, we observe alarming patterns in data quality, which adversely affect outcomes of analytics and sometimes make these outcomes incorrect resulting in missed operational KPIs, lost revenue, and reduced quality of care.
Below are a few of the key patterns signifying poor data quality that we’ve observed over the years.
Poor Data Governance at the Source
The implementation of a modern EHR is a massive undertaking involving vendor and third-party consultants who work on configuration and customization of the EHR modules at each of the organizational units. Different consultants often configure different modules of the systems independently which results in duplication or overlap of data elements they create. Such conflicts and duplication don’t affect the functions of individual units but may adversely affect analytics performed across the entire organization. As an example, we discovered seven Length of Stay (LOS) metrics at one of the major Health IDNs running on Epic EHR. These differing definitions for LOS can create multiple types of inaccurate reporting if the various definitions are not accounted for.
ETL Processes Always Result in the Loss and Distortion of the Data
Analytics is usually performed on the data in reporting databases or data warehouses. These repositories aggregate data from one or more operational sources by means of ETL processes (Extract, Transform, and Load). As part of these processes, some of the details of the original data may be lost or distorted. The resulting data often loses original structure, granularity, and traceability to the source. Some of the relationships with other data elements may be lost as well.
Flawed Data Mapping
Transformation and aggregation of clinical data is a complex medical informatics task involving mapping and reconciling vocabularies and clinical concepts which are usually represented differently in the source and destination data schemas. Yet, the development of ETL processes is often performed by IT personnel without the appropriate involvement of trained clinicians and/or medical informaticians. Moreover, given that patient data is constantly changing, coded terminologies and classifications evolve and undergo revisions, the data mapping must be subjected to continuous realignment, without which the quality of reporting data will deteriorate over time.
Lack of Standard Coded Concept Tagging
It is believed that structured data is easier to analyze reliably than unstructured (free form) data. This is generally true, but even well-structured data may have little value outside of the source system within which it was created. When data needs to be compared and mapped against data from multiple systems tagging with standard medical terminology and classification concepts is crucial. Billing data would have been nearly meaningless to insurance companies without ICD codes attached to diagnoses. EHR systems would have had difficulties communicating with laboratories without LOINC codes. However, many other types of data elements in the extracted data sets are often lacking standard coded concept identifiers making it difficult to map, reconcile and analyze across multiple data sources.
Importance of Metadata for Analytics
Metadata (auxiliary information about the data) is highly valuable when it comes to analytics. Metadata includes such elements as ownership, change history, the context within which the data was created. Among other benefits, metadata allows reconstructing relationships between data entities in time and in sequence. An inpatient order may contain the id of the provider who placed the order but usually not the role (e.g. attending, responsible clinician) of that provider at the time the order was placed.
Much like the data itself, the metadata is often lost or distorted during data extractions and aggregation. However, some of the metadata can be reconstructed and even enriched from other entities and alternative data sources.
Making Clinical Analytics More Meaningful and Accurate
As we’ve learned to identify the symptoms of deficient data, we’ve also developed our own strategies for improving data for reporting and analytics – before, during, and after the extraction, aggregation, and reconciliation efforts.
Working with clients – from Academic Medical Centers to innovators in life sciences and clinical research we observe how these strategies bear fruit – bringing high-quality actionable insights directly to the patient bedside, driving improvement initiatives, predicting performance for value-based care, and improving patient outcomes.