How Operational Healthcare Technologies Can Improve Clinical Research
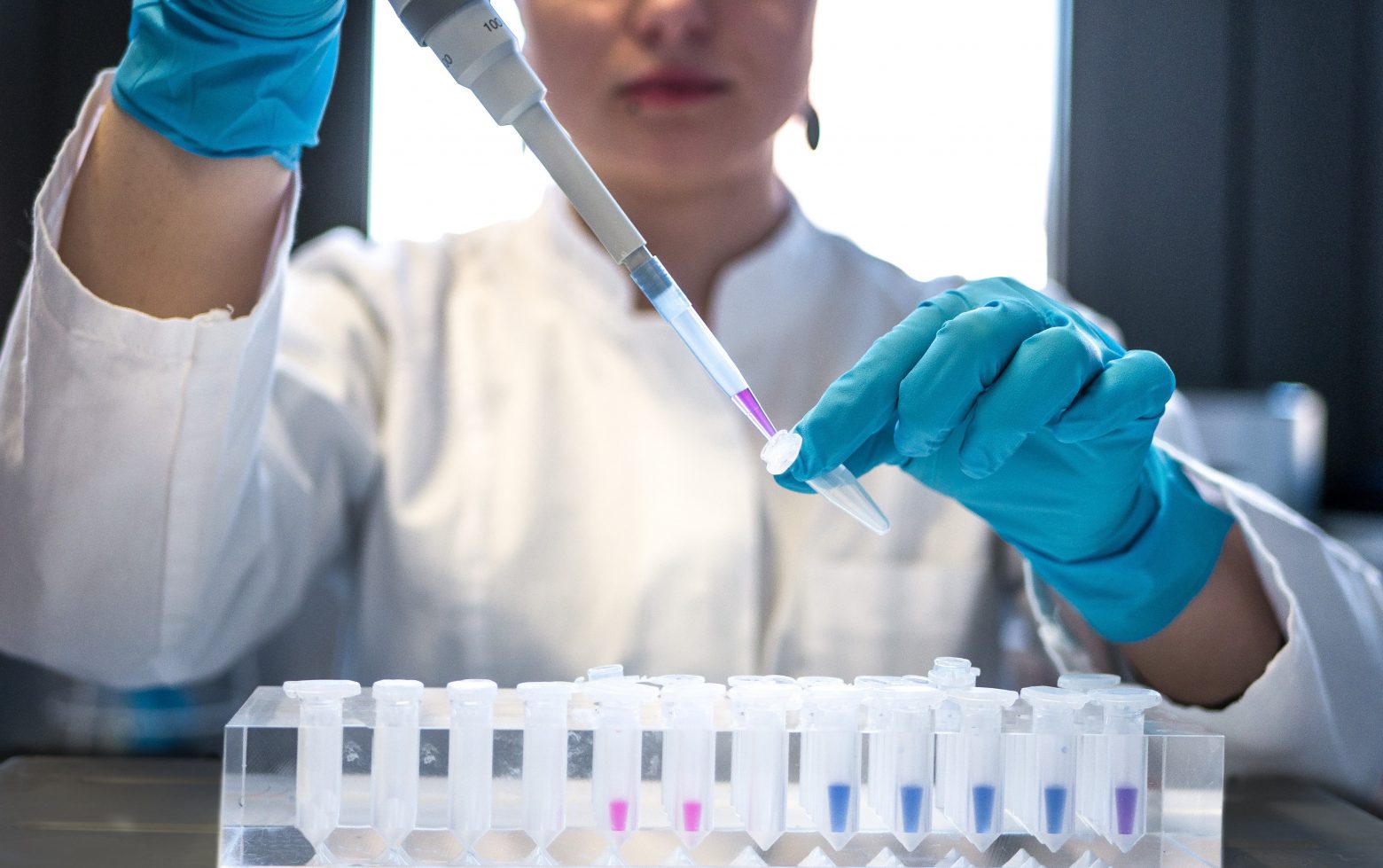
Evidence-based clinical research is one of the essential innovations in Healthcare and Life Sciences of recent years. Rather than relying on information collected as part of individual studies and clinical trials, evidence-based research is taking advantage of the vast amount of patient data now available in EHRs and other systems generated as part of routine care.
Health institutions and research organizations often team up into research networks, often identified as Shared Health Data Networks (SHDNs), that standardize on a specific Clinical Data Model (CDM). One of the primary goals of research networks is to make a larger population of patients from participating institutions available for research.
There are several competing and complementary CDMs in existence. These CDMs are created, sponsored, and supported by open source communities, governmental organizations, and private entities.
Informatics for Integrating Biology and the Bedside (i2b2) is one of the CDMs that was initially created at Partners Healthcare in Boston (now Mass General Brigham) for the research data warehouse called the Research Patient Data Registry (RPDR) and subsequently released into open source. In 2017 i2b2 Foundation combined forces with the TransSMART foundation. Today, the i2b2 tranSMART Foundation is integrating its two leading information and analysis platforms in clinical research and translational science.
Observational Medical Outcomes Partnership (OMOP) CDM was initially published in 2007. The Observational Health Data Sciences and Informatics (or OHDSI, pronounced “Odyssey”) is a multi-stakeholder, interdisciplinary collaborative standardized on OMOP.
In many of its activities, European Health Data & Evidence Network (EHDEN) utilizes OMOP and collaborates with OHDSI.
Other examples include the Patient-Centered Outcomes Research Institute (PCORI) CDM that powers SHDN called PCORnet, The National Patient-Centered Clinical Research Network, Sentinel — a CDM used by Sentinel Data Partners, and many others.
Network participants conduct “eligibility queries” to identify cohorts of patients for a specific study or trial. Finding cohorts of patients eligible for a specific study or trial is a multistep process involving the definition of multiple hypotheses for patient eligibility and conducting multiple eligibility queries against available data sets in CDM.
In the majority of cases, organizations rarely freely contribute their patient data to a single common CDM repository (Pooled SHDN). Instead, organizations construct their local repositories based on CDM and expose them in a federated, distributed data network (Federated SHDN), allowing participants to execute limited and controlled types of queries.
Standardizing on a common CDM allows health care providers to share patient data securely while ensuring that all participants have access to the same information.
The process typically involves extensive data quality analysis and mapping source data structures and concepts into a single target representation. The mapping process may involve assigning or mapping coded identifiers, reconciling vocabulary, and other steps.
The quality, richness, and usefulness of the data in CDM are very much dependent on the level of expertise of the team that conducts the ETL. Many teams have produced inferior quality CDM data due to a lack of knowledge in extracting accurate, reliable information from their source systems.
Few research organizations have significant experience and capacity to set up and conduct ETL processes with the right quality and effectiveness. In most cases, researchers utilize open source tool sets to perform the ETL and conduct eligibility queries. Such tools are often relatively primitive — simple scripts developed by individual researchers.
On the other hand, similar data extraction and transformation services are performed as part of operational systems integration efforts involving complex workflows and many heterogeneous systems – EHRs, labs, radiology systems, payor systems, and many others. Many commercial organizations working in Healthcare have developed sophisticated workflow orchestration and data transformation technologies for building such data integration pipelines.
While the end goals for ETL in clinical research and Healthcare operations differ, there is an opportunity to utilize the same technological toolbox for both. Recognizing the opportunity, leading commercial vendors in Healthcare, like InterSystems, have begun to offer low-cost or open-source variations of their commercial solutions to research organizations.
The advent of sophisticated commercial tools has made it possible not only to optimize and improve quality within the traditional research ETL processes and workflows. They also open the door for other opportunities where CDMs could play a major role – such as cross-institutional Clinical Decision Support, incremental ETLs, and improved operational analytics. It is only a matter of time before the research communities fully embrace the technologies and support of commercial vendors.
Learn more on First Line Software’s Healthcare Practice