Ensure Your GenAI Models Meet the Highest Standards
Explore our full range of GenAI evaluation services, designed to guarantee accuracy, safety, and reliability
Explore servicesBoost GenAI Performance with Advanced Tools
See how our cutting-edge tools enhance the reliability, scalability, and efficiency of your GenAI models.
Explore the toolsProven Methods for Accurate GenAI Evaluation
Learn about our tested techniques to ensure your GenAI models are safe, reliable, and free from risks.
See our processReady to Secure and Optimize Your GenAI?
Get expert guidance tailored to your needs. Submit your request today to begin your GenAI evaluation.
Submit your requestConcerned About GenAI’s Behavior With Customers?
Keep Your Model Under Control.
Concerns About Bias and Fairness
Validating Model Accuracy and Reliability
Compliance with Industry Regulations and Ethical Standards
Evaluating Model Performance Across Different Scenarios
Evaluating Model Security and Privacy Risks
Let’s work together to mitigate your risk, so you can start to realize the return on your investment faster.
Speak to an expertGenAI Evaluation Services
GenAI Evaluation and Quality Assurance
A comprehensive assessment of GenAI models to ensure accuracy, reliability, and safety. Includes the identification and mitigation of biases, hallucinations, and the development and application of tailored evaluation metrics.
Risk Management
We proactively identify and assess the risks associated with GenAI systems. Includes the development and implementation of risk mitigation strategies, and ongoing monitoring and response to emerging risks.
Optimization and Improvement
The continuous evaluation of GenAI models to identify performance bottlenecks, and the implementation of optimization techniques to enhance model accuracy and efficiency.
Dataset Development and Management
The creation and curation of high-quality datasets for training and evaluating GenAI models. Includes ata cleaning, labeling, augmentation to improve model performance, and data governance and privacy compliance.
Tooling and Infrastructure
The selection, implementation, and optimization of AI tools and platforms. We handle design and deployment of scalable AI infrastructure as well as the integration of AI into operations for efficient workflows.
AI Strategy and Consulting
The development of tailored GenAI strategies aligned with business objectives.
Accelerated GenAI Excellence
First Line Software has been a leader in the most advanced ways of software development for decades. Our engineers, who pioneered the significant milestones of Agile, Public Cloud, and DevSecOps, now lead GenAI-empowered software development. By adopting this cutting-edge technology into our processes, First Line Software’s teams develop exceptional software faster, while maintaining top-level quality. We constantly adopt the best GenAI tools available, as well as create our own GenAI tools to accelerate our client’s technology excellence and shorten their time-to-market. That means our clients see better results, sooner.
GenAI is a revolutionary productivity amplifier, and we can show you how it can work for your business. The GenAI marathon has already started- let us help you set the pace. We aim to find the perfect, custom fit for your company for the long haul.
How We Work
During the evaluation process, our AI QA Engineers employ various methods to evaluate GenAI solutions:
Utilizing Human-Created Datasets:
Leverage datasets created by humans that encompass specific domain knowledge, biases, security aspects, and more to evaluate different facets of GenAI-based solutions.
Employing LLM Models with Human Review:
Use Large Language Models (LLMs) to generate datasets, which are then used to thoroughly evaluate GenAI applications under supervision of an AI expert.
Our Tools
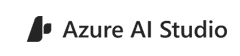
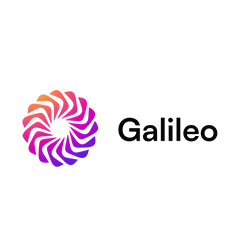
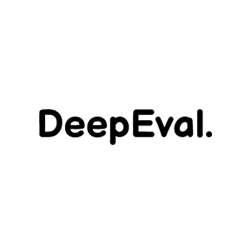
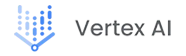
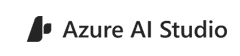
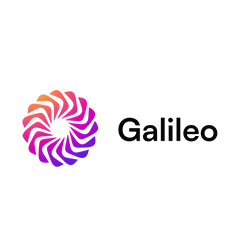
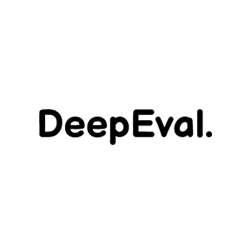
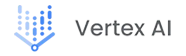
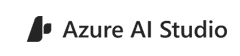
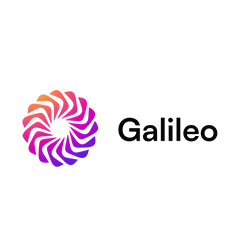
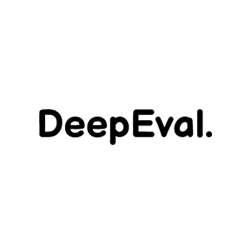
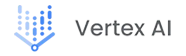
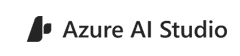
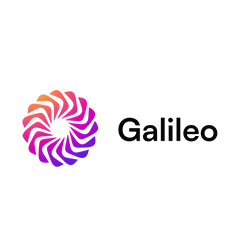
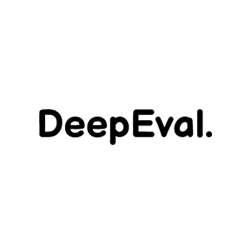
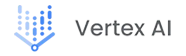
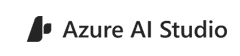
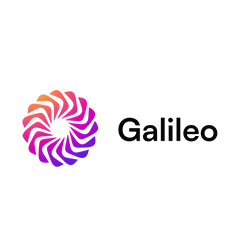
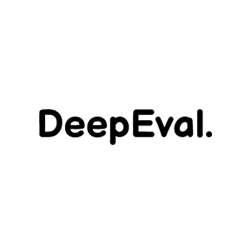
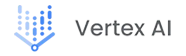
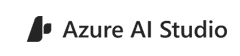
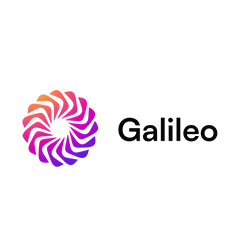
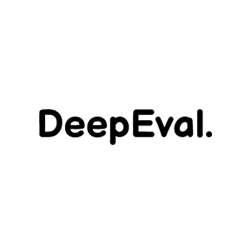
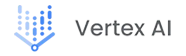
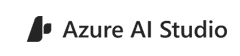
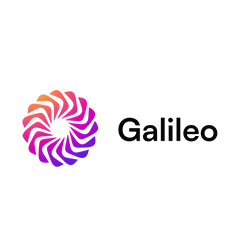
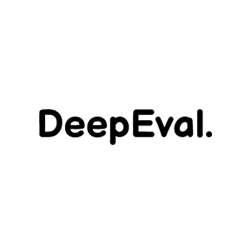
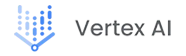
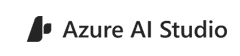
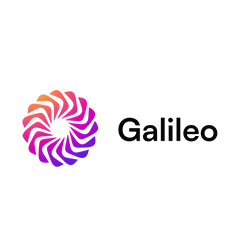
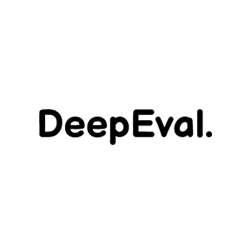
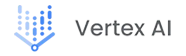
GenAI has revolutionized software development, offering endless possibilities for engineers. This shift challenges traditional quality assurance methods, but pioneering work in data science and cybersecurity has led to new approaches. We’re thrilled to introduce GenAI Evaluation, a vital part of this new frontier, aimed at equipping individuals with the knowledge needed to navigate this exciting technology.”
Pavel Khodalev
SVP, Global Business, First Line Software
Clients Love Us
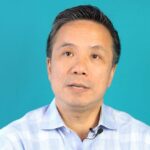
Qi Li
Physician Executive, Product Development At Intersystems
“They are very good at understanding the requirements but more importantly they can think about the future requirements and future proof your project.”
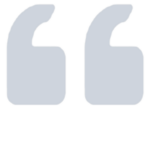
Executive
Real Estate Appraisal Company
“First Line Software is paying attention and proactively managing the project with us while doing extra things that go above and beyond what we expect.”
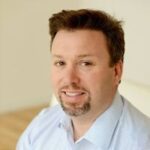
Jay Thomas
Chief Strategy Officer, Triptych, USA
“First Line Software has a large team, and because of the breadth of services, they can give you that flexibility to work on other projects. Adding another technology to your stack, for example, is one way they could support you.”
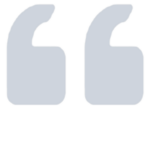
Lauren Tindall
Technology Project Manager, Sandy Alexander, New York
“I’ve worked with other developers, but I found that the First Line Team is very knowledgeable and helpful. I would totally recommend them to anyone who’s looking into their services.”