Securing Your Generative AI Solution: Risks, Compliance, and the Path Forward

Principal Engineer
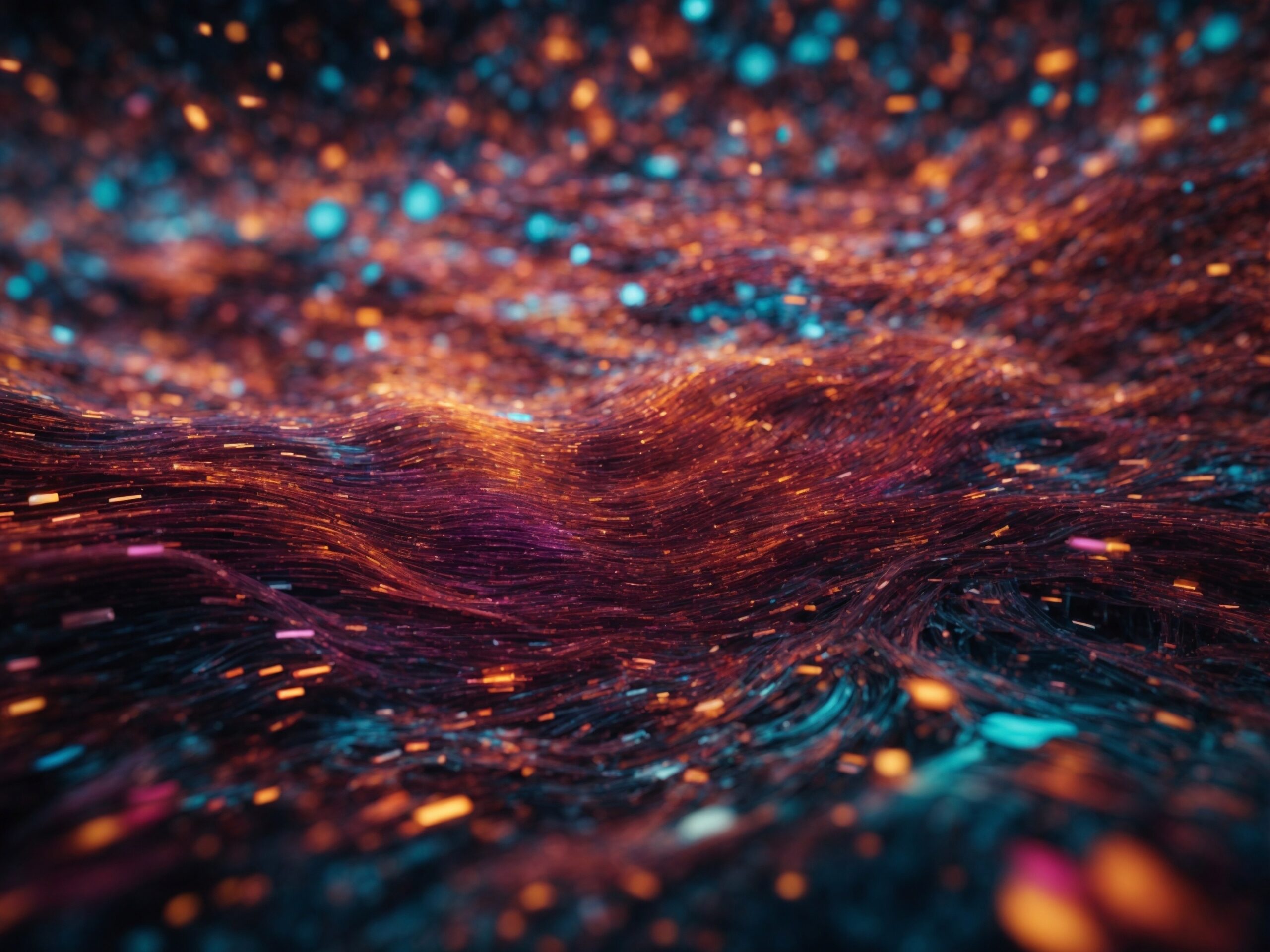
This article explores the critical considerations for securing your generative artificial intelligence (GenAI) solutions. As GenAI adoption spreads across business sectors, organizations need to prioritize security practices that mitigate risks and ensure compliance.
Why Gen AI Needs Security
GenAI has immense potential for various applications. However, these powerful tools also introduce new security challenges. The ability to process and generate vast amounts of data necessitates a comprehensive security strategy to protect sensitive information and prevent misuse.
History Repeats Itself – Security Cannot Be Ignored
The information security (InfoSec) industry has a history of overlooking security during the initial phases of adopting new technologies. This trend continues with generative AI, where security is often an afterthought.
However, generative AI is not fundamentally different from other technologies from a security perspective. By implementing and adapting existing security controls and best practices, organizations can effectively safeguard their GenAI solutions.
Whose Data Is It Anyway?
Generative AI models often rely on substantial datasets, which may include sensitive company information. This raises crucial data security questions:
- Does an individual have the authorization to share the data they access?
- How does the Gen AI system utilize the data it receives?
Organizations should conduct thorough evaluations to ensure their existing data security controls effectively extend to generative AI implementations.
AI and Information Security
The core principles of information security remain paramount when working with Gen AI:
- Principle of Least Privilege: Grant Gen AI systems access only to the data strictly necessary for their tasks.
- Data Encryption: Encrypt data at rest and in transit to safeguard sensitive information.
- Access Controls and Need-to-Know: Implement robust access controls and enforce the “need-to-know” principle to restrict access to authorized personnel.
- Logging and Auditing: Maintain meticulous logging and auditing practices to monitor access and identify potential security incidents.
Generative AI should be subject to the same rigorous security standards as any other automated system. The data used to train a model answering customer product manual inquiries should not include company contracts or financial data, for example.
Furthermore, organizations should acquire the necessary compliance and security approvals for generative AI solutions, facilitating proper auditing and risk management.
The Foundation Model Needs Security Too!
When building a solution around a generative foundation model, the solution itself should incorporate security features. These features may include input validation and training the model to identify and counter malicious actors.
For instance, a solution providing customer product manuals should be equipped to prevent bad actors from manipulating the model into delivering incorrect or misleading information.
The entire software development lifecycle (SDLC) for a generative AI solution deployed for business purposes should be auditable to demonstrate adherence to secure coding practices. This is equally important for both the foundation model itself and the implementation layer surrounding it. Interestingly, generative AI can be leveraged within the SDLC to enhance the security of the solution itself.
Do I Own What My Foundation Model Solution Creates?
The legal implications surrounding the ownership of outputs generated by foundation models trained on potentially non-proprietary data are a significant concern. Consulting with legal counsel is crucial for organizations navigating this complex legal landscape.
Currently, the legal framework around ownership of Gen AI outputs remains largely undefined. While some foundation model owners may grant copyright permission, the validity of such copyrights may be contingent on the legal jurisdiction and the data used to train the model.
Organizations leveraging Gen AI solutions must be aware of the potential copyright infringement risks associated with the model’s outputs. In the United States, for example, the Copyright Office does not grant copyright protection to works solely generated by AI.
The line between human and AI contribution in the creation process remains a moving target, and legal precedents will likely evolve alongside the technology. Organizations should closely monitor legal developments in this domain.
Are There Other Risks?
Generative AI solutions necessitate additional layers of monitoring and logging beyond traditional infrastructure and software audit trails. It is crucial to log and track queries and the corresponding generated responses to ensure performance, and accuracy, and mitigate potential social concerns arising from language misuse or misinformation.
How Can I Use Generative AI to Make My Company More Secure?
Generative AI presents opportunities to enhance an organization’s security posture. Here are some potential applications:
- Integration with Security Technology: Generative AI can be integrated with existing security tools, such as simulating cyberattacks to identify vulnerabilities and training security personnel on the latest attack methods employed by malicious actors.
- Proactive Security Solutions: Generative AI can be utilized to develop proactive security solutions, such as generating synthetic data for application security testing or identifying previously undetected anomalies within historical security logs.
Conclusion
Generative AI offers a transformative landscape of possibilities across various industries. However, to unlock its full potential, organizations must prioritize robust security practices. This white paper has explored the critical considerations for securing generative AI solutions, emphasizing the importance of data security, access controls, and compliance. By adhering to established security principles, leveraging generative AI for security testing, and staying informed about evolving legal considerations, organizations can navigate the path forward with confidence. As GenAI technology continues to mature, a commitment to security will be paramount in ensuring its responsible and beneficial implementation across the business landscape.